
Creating AI Tools To Identify High-Risk COVID-19 Patients
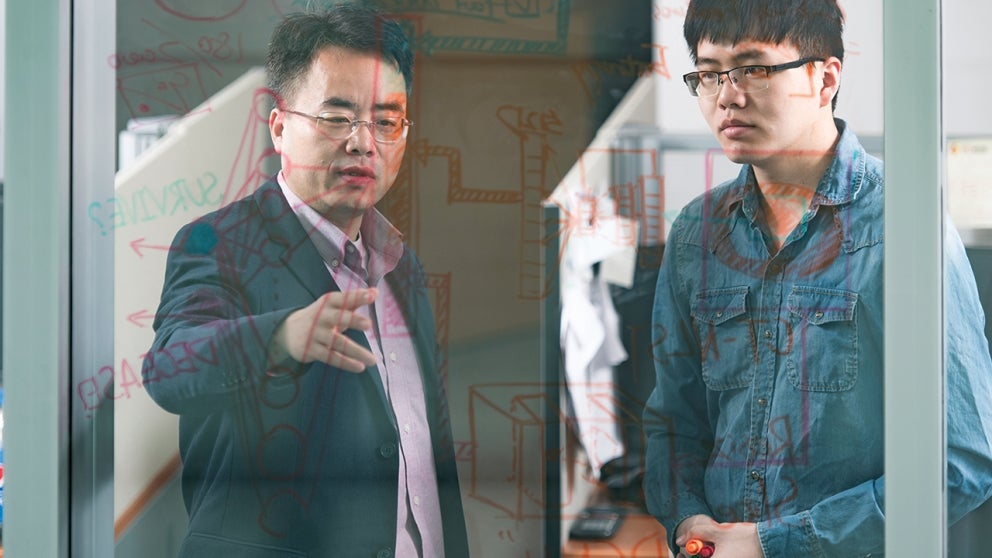
Improved screening and treatment options for COVID-19 are critically important for high-risk patients with comorbidities, such as diabetes, pulmonary disease, and cardiovascular disease.
A new grant from the National Institutes of Health (NIH) will support the rapid development and integration of a series of artificial intelligence algorithms that will analyze multiple pieces of health data — from chest computed tomography (CT) images to vital signs — in order to help clinicians assess disease severity and predict patient outcomes. The effort is being led by Pingkun Yan, assistant professor of biomedical engineering.
“Screening out the high-risk patients who may need intensive care later, and monitoring them more closely to provide early intervention, may help save their lives,” says Yan.
According to Yan, the few artificial intelligence tools that are currently available in the COVID-19 fight can only help doctors determine the severity of lung infection brought about by the disease. They fail to assess and account for comorbidities.
The research team will create a framework of algorithms that can integrate critical information about a patient, including CT scans that assess the severity of lung infection, patient demographic information, vital signs, and laboratory blood test results.
“My group has been focusing on using artificial intelligence and deep learning to analyze medical imaging data with an emphasis on translating the technology from benchside to bedside,” Yan says. “This focus built a solid foundation for us, so when the crisis emerged, with our clinical collaborator at Massachusetts General Hospital, we quickly identified the clinical needs and started working on a solution.”
In partnership with Massachusetts General, this NIH-supported research will be fast-tracked, with the hope of implementing the team’s algorithms as quickly as possible.