
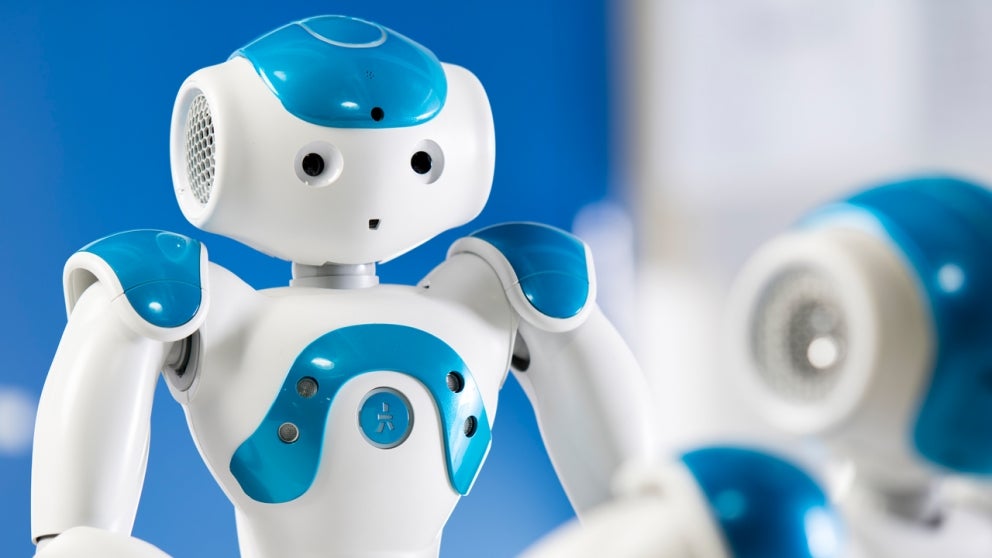
Delving Deep Into Artificial Intelligence
Contributors: Jodi Ackerman Frank and Mary Martialay
Rensselaer is at the forefront of AI research and education. World-renowned faculty — working with new tools, infrastructure, and world-class environments — are pushing the boundaries of exploration in AI.
Artificial intelligence (AI) is everywhere. It helps us navigate our daily computer searches for work, school, and leisure time. It serves as the basis of the mesmerizing efforts to make cars, trucks, and drones fully autonomous.
It is used in computer simulations to enhance the human experience and benefit society at large, including gaming and learning in new ways within a three-dimensional computer-human environment. AI is expected to revolutionize the health care and financial industries, the way we garner new knowledge about climate change, and even in the efforts to develop an autonomous electric grid.
Groundbreaking work is being conducted in labs and classrooms across the campus. Rensselaer researchers, as well as students, are collaborating in some of the most advanced platforms in the world. These facilities and spaces include the Cognitive and Immersive Systems Laboratory (CISL), a multiyear collaboration with IBM Research that is leading the frontier of research and development in immersive environments.
Another partnership — the Artificial Intelligence Research Collaboration — is a multiyear effort between Rensselaer and IBM that involves multiple graduate students, postdocs, research scientists, and faculty working closely with IBM researchers to push the frontiers of AI research and apply their results to some of the world’s key global challenges.
Recently, Rensselaer was named a partner in a new $2 billion IBM Research AI Hardware Center, designed to further accelerate the development of AI-optimized hardware innovations.
And there are numerous researchers using AI to tackle problems in a variety of fields. In his Sensing, Estimation, and Automation Laboratory, John Christian, assistant professor of mechanical, aerospace, and nuclear engineering, is using it to help spacecraft navigate the solar system more effectively. Rich Radke, professor of electrical, computer, and systems engineering (ECSE), is working to develop conference rooms that can actually facilitate meetings. Fellow ECSE professor Qiang Ji is using AI to enable robots to recognize human behaviors and emotions, and to interact with them more naturally.
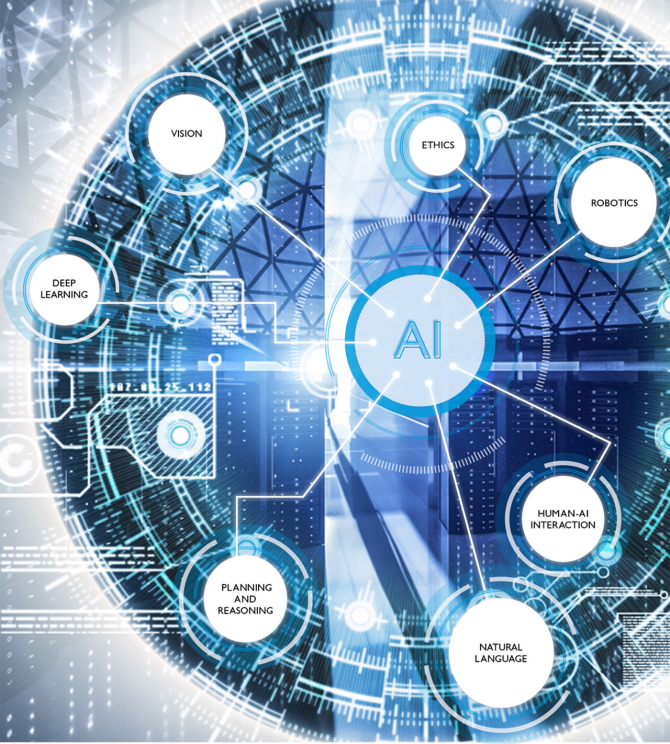
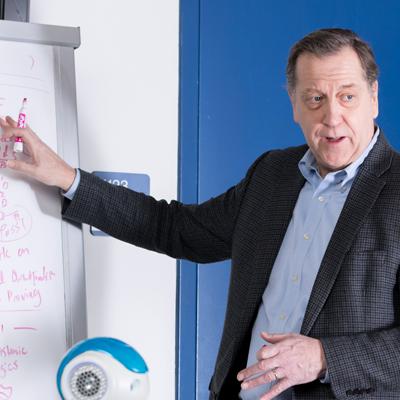
Pushing Boundaries, Exploring Limitations
Selmer Bringsjord, whose research spans several disciplines, including AI, cognitive science, and computing, is conducting groundbreaking humanoid research in the lab he directs, the Rensselaer Artificial Intelligence and Reasoning (RAIR) Laboratory.
RAIR uses robotics and computational capability platforms for researching applications of reasoning.
“The RAIR Lab compels overall society to ask fundamental questions about AI,” Bringsjord says. “For instance, will machines ever mimic the complexities of the human mind?”
In working toward that long-term end goal, Bringsjord has led teams of students and researchers in the development of tabletop robots with initial levels of self-awareness.
“These humanoids are able to sense and to reason, and because of it, to pass a new and difficult test of machine self-awareness,” Bringsjord says.
In this test, three robots were programmed to understand that two of them had been given a special “dumbing pill” (activated by a push of a button) that would not allow them to speak. The third one received a placebo. The one who could still speak had to figure this out on its own, which it did once it heard its own voice. Initially, one robot responded, “I don’t know,” when asked which of them received the pill. Then, upon hearing its own voice, it realized that it had not been silenced by the dumbing pill, and answered correctly.
Bringsjord is also exploring whether ethics can be engineered into robots as part of a multimillion-dollar AI project. The project, funded by the U.S. Navy, is a collaboration among researchers from Rensselaer, Tufts University, and Brown University to develop autonomous robots that can make automatic ethical judgments on their own when faced with ethically “thorny” circumstances, which often occur on a battlefield.
“We’re talking about robots designed to be autonomous — meaning you don’t have to tell them what to do,” he says. “When an unforeseen situation arises, a capacity for deeper, on-board reasoning must be in place, because no finite rule set created ahead of time by humans can anticipate every possible scenario.”
Still, in all the AI innovation taking place at Rensselaer and elsewhere, the field of AI has a long way to go to mimic humans in even the simplest of ways.
“In our enthusiasm and quest for AI technologies, we have to be aware of how little machines can really do compared to the human brain,” says Bringsjord. “Even a tiny child knows that the feelings of others ought not to be be trampled, but a machine doesn’t have feelings, and hence can’t empathize.
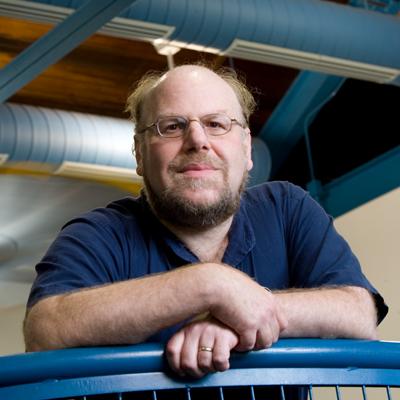
James Hendler, a renowned expert in the Semantic Web, artificial intelligence, and agent-based computing, agrees. Hendler is the director of the Rensselaer Institute for Data Exploration and Applications (IDEA).
“The AI advancements that are now beginning to enter into mainstream societal use — speech recognition, natural language chatbots, improved computer vision systems, the things that are coming from deep learning systems — work very well in a narrow context,” Hendler says. “But when you try to stretch them into a new situation, they break down.”
In other words, AI technologies are able to meet or exceed human performance in areas that exploit the computer’s large memory, mathematical algorithms, and various search techniques. But there is a vast gulf between the current capabilities of AI and the omniscient machines envisioned in science fiction.
For computers, understanding the world we live in, comprehending the historic and social contexts of situations, and using common sense reasoning are still daunting obstacles.
“These are not necessarily insurmountable hurdles,” Hendler adds. “In fact, a more pervasive, seamless interaction with AI is inevitable.”
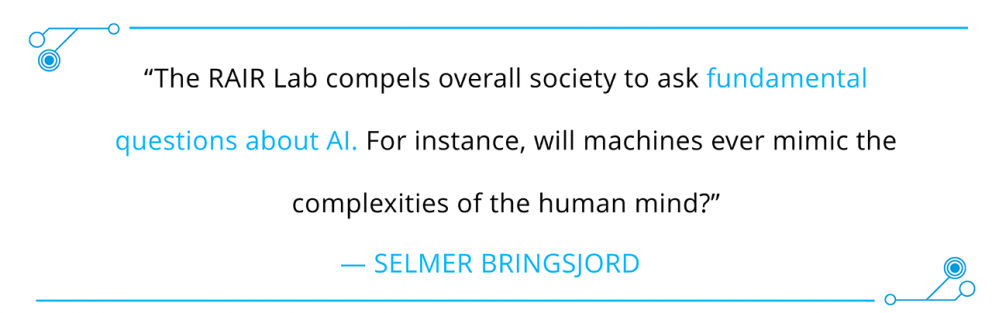
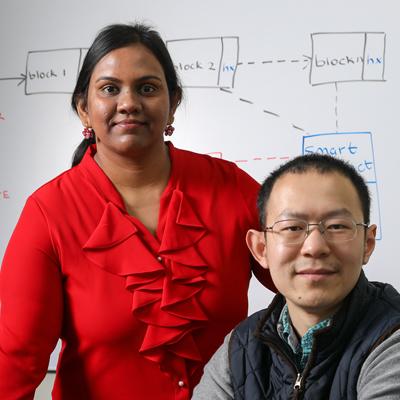
Autonomous Transactions
Despite major hurdles to overcome, it is fairly clear that AI is expected to transform health care as we know it.
For their part, computer scientists Oshani Seneviratne and Lirong Xia are focusing on the world of blockchain smart contracts to create new ways to improve patient health care and communications.
A “blockchain is nothing more than a data structure — a block of data — that enables a transaction ecosystem,” Seneviratne says. “It is a bulletproof mechanism to hold people accountable for whatever agreement they’ve made.”
Seneviratne is the director of health data research for the Health Empowerment by Analytics, Learning, and Semantics (HEALS) project.
HEALS is a five-year collaborative research initiative that exploits innovative capabilities in cognitive computing, coupled with human behavior, smart-device development, and semantic data analytics to solve the world’s biggest health-care challenges in diagnosing disease, making accurate prognoses, and addressing other multifaceted patient care concerns.
The blockchain was invented to support cryptocurrency, but Seneviratne and Xia are interested in its applications in health care. This security facilitates transactions that would ordinarily rely on traditional market safeguards, such as established reputation or a centralized authority.
Blockchain may one day enable AI agents to autonomously arrange maintenance in a smart home, trade in products — such as prescription drugs — in which provenance is important, or arrange for the transfer of sensitive information — like your health records — between appropriate parties.
“Anything that can be written as a contract can be recorded in a blockchain, and applications built to trade autonomously based on that contract would only complete a transaction if it adhered to the terms coded in the blockchain,” Seneviratne says.
Once organized, the block is sealed with a computational lock that is based on the previous block, and that lock is in turn used to generate the lock for the next block in the chain. To break into any given block, one would have to unpackage all the later blocks in the chain, which, given computational hurdles imposed by blockchain protocol, is not currently possible.
The strength of the blockchain is that, once coded into the chain, the contents of a contract can be shared among peers but are nearly invulnerable to alteration. This means transferring health records could benefit immensely from the power of blockchain to reduce inefficiency and red tape.
Imagine a future, made possible by the applications Seneviratne is helping to build, where automatic access to your health records is brokered by contracts protected by blockchain. AI agents would move necessary information between you, your doctors, and insurance companies, eliminating delay and inefficiency.
She is also testing approaches to autonomous solutions, and she envisions a future where the impenetrable security of blockchain would combine with a new flexibility to respond to unanticipated contingencies.
For example, if you are involved in an accident and sent unconscious to an emergency room in which doctors don’t have immediate access to your medical records, how could an AI-based system autonomously determine whether to provide them?
“We’re trying to figure out how we would handle and execute such unknowables in the future,” she says.

IBM Research and Rensselaer are collaborating on a new approach to help students learn Mandarin. The strategy pairs an AI-powered assistant with an immersive classroom environment that has not been used previously for language instruction. The classroom, called the Cognitive Immersive Room (CIR), makes students feel as though they are in a restaurant in China, a garden, or a Tai Chi class, where they can practice speaking Mandarin with an AI chat agent. The CIR was developed by the Cognitive and Immersive Systems Lab (CISL), a research collaboration between IBM Research and Rensselaer.
When learning a new language, especially one as difficult as Mandarin, it’s important that students have many opportunities to speak and practice their conversational skills. Acquiring a new language naturally, through cultural immersion, may be more effective than non-immersive practices.
The CIR brings together several state-of-the-art technologies such as speech-to-text, natural language understanding, and computer vision to enable immersion and natural multimodal dialogue. The room includes a 360-degree panoramic display system, an audio system, multiple cameras, multiple Kinect devices, and multiple microphones, as well as computer systems to support the AI technologies, some located in the room and others in the cloud.
“Our goal is to combine cognitive, immersive technologies with game-playing elements to enable students to experience a cultural environment, practice daily tasks, and get help from intelligent agents,” says Hui Su, who leads CISL. “With the Mandarin Project, we use IBM Watson within the CIR as a conversational agent to engage students while they learn the language. Our approach involves IBM Watson speech recognition and natural language understanding technologies for English and Chinese.”
Excerpted from a post in the IBM Research blog.
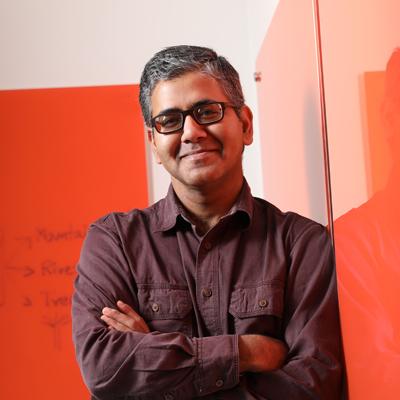
Mimicking Neural Networks
Mohammed Zaki, professor of computer science and a project lead on the HEALS team, is also hoping to imbue AI with greater flexibility through associative memory, in which a network of artificial neurons or a neural network stores interconnected facts and relationships for later recall with partial cues. Neural networks are computational constructs that mimic the web of interconnected neurons found in biological brains. The idea of neural networks was conceived long before technology was up to the task, and Zaki says the concept is now experiencing a resurgence.
If you wanted to look up Mohammed Zaki, but misspelled his name, for instance, a computer might not find him.
“Computers basically store and retrieve data from databases — cells, columns, and rows,” he says. “And we retrieve it using a structured query. But we can only return exactly what we’re looking for. And if you don’t know what you’re looking for, or if the data gets corrupted, then it’s pretty much useless.”
“Humans, however, are very good at what we call ‘associative recall,’” Zaki adds. “You think of one thing and it triggers a memory. One piece triggers another, and humans can trigger a pathway from a small part to a whole, and from one aspect to all sorts of unexpected associations. Our computer systems are unable to tackle this kind of retrieval.”
Neural networks are commonly known for their success in pattern recognition. When you hear about computers being “trained” to recognize faces, or speech, or tumors, chances are neural networks are involved.
Using a technique called deep learning, artificial neural networks can extract and cluster the relevant features of the input they are being trained to recognize. So, how would this work if you were still trying but struggling to look up “Mohammed Zaki”? To begin, he wants to encode simple associations into the network — Mohammed, Zaki, Rensselaer, professor, computer science.
To do this without changing the simplicity of artificial neurons, Zaki must make it possible for the neurons to store data and change how neurons are connected, the weights between them, and the levels of layers used to process a query, from granular to abstract.
The computer would now contain a network of features that define “Zaki,” a network that could be retrieved with a variation on the theme, and potentially retrieve other webs connected to the theme. Due to the “memory” being distributed throughout the neural network, we can still retrieve relevant facts even if parts of the network get damaged.
Such capability would be a potent tool in health care, which is his principal application. And a system that begins to have a representation of “Zaki” may also have the capacity to develop representations of abstract concepts that even humans have a hard time defining, such as “justice” and “freedom.”
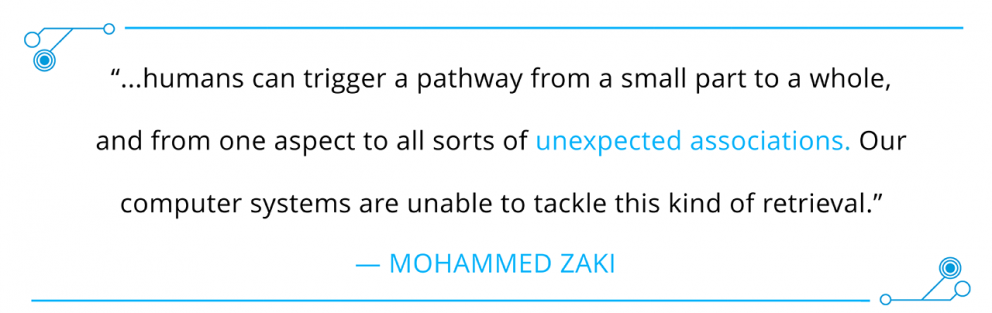
Immersive Environments
Major Rensselaer platforms and centers, such as CISL, are enabling researchers to combine advances in artificial intelligence and cognitive computing, using sensor- and actuator-rich immersive technologies.
“The goal is to vastly improve group decision-making — in many different fields,” says Hui Su, who leads CISL.
CISL bridges human perception with intelligent systems in an immersive, interactive setting — enabling environments such as a cognitive design studio, boardroom, medical diagnosis room, or classroom.
The lab supports the groundbreaking Mandarin Project, the first of many “Situations Rooms” to be developed that uses mixed reality and immersion, as well as a semester-long multiplayer game, to teach Mandarin Chinese.
PAGI World (“pay-guy”), which stands for Psychometric Artificial General Intelligence World, is another simulation platform, which allows AI researchers and students to test out their ideas on a wide variety of tasks, and also to create their own tasks, in a real-time environment with realistic physics.
Many real-world elements have been created within this platform that don’t exist in other simulation environments, such as those based on water/fluid dynamics and vision. The program also allows AI agents to be written in virtually any programming language.
Another contributor includes the Collaborative Research Augmented Immersive Virtual Environment Laboratory, or CRAIVE-Lab, headed by Jonas Braasch, professor of architecture and expert in architectural acoustics. It already is being used by architecture students for human-scale group design.
To further support discussions among small groups of people, Senior Research Engineer Eric Ameres of EMPAC has developed something called Campfire — a virtual firepit that serves as an interactive interface for visual information, representation, and collaborative analysis.
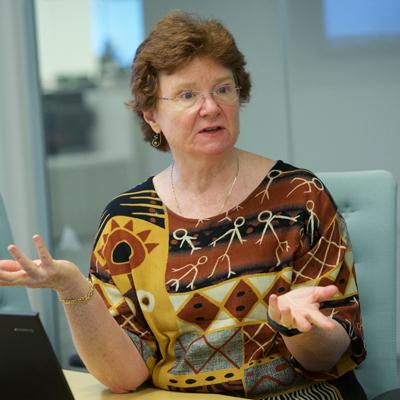
Language Models
Such cyber-physical interactions require a laborious handcrafting of the topics and the language model. Hendler has joined forces with Heng Ji, the Edward P. Hamilton Development Chair Professor, and Mei Si, associate professor of cognitive science, to tackle this area.
The idea is to automate the creation of cognitive agents able to use “living information extraction,” which will, among other things, pull information from the Web about entities and events, analyze the relationships between them, decide what is interesting about them, and use storytelling techniques to present the information to the user.
Deborah McGuinness, Tetherless World Senior Constellation Professor, who plays an integral role in Rensselaer IDEA research, is also a leading expert in machine knowledge representation and reasoning languages, which are cornerstones in the development of the Internet of Things (IoT).
AI is crucial in the development of the IoT, a wireless infrastructure that connects devices and systems, from our phones and kitchen appliances to roads, vehicles, and the electric grid, to obtain and share data, adapting to changing conditions along the way.
McGuinness is developing knowledge bases of both metadata and data for initiatives such as the National Science Foundation’s Ontology-Enabled Polymer Nanocomposite Open Community Data Resource and previously for the Jefferson Project at Lake George. Metadata, essential for realizing the big data potential in IoT, interpret, integrate, and determine relationships among all the basic data generated from research at various times and locations. Data resource websites allow users to share and reuse the data across applications, enterprises, and community boundaries.
McGuinness is one of the founders of an emerging area of semantic eScience — introducing encoded meaning, or semantics, to virtual science environments. Within this intersection of artificial intelligence and eScience, she incorporates semantic technologies in a range of health and environmental applications.
In one epidemiology project, McGuinness is leading the data science efforts of the Child Health Exposure Analysis Repository in collaboration with the Icahn School of Medicine at Mount Sinai to advance the understanding of environmental exposure on children’s health and development.
The data center is one of three components funded by the Children’s Health Environmental Assessment Resource (CHEAR), a program established by the National Institute of Environmental Health Sciences.
A large part of her role is to develop foundation and methodologies for combining data from a wide range of environmental health studies. These methodologies include creating precise vocabularies and ontologies for use in integrating the data and ontology-enabled services.
Ontologies define a set of concepts and the relationships among them. For example, the term “infant” is not defined the same as “child,” but could be considered a subclass of “child.” The same holds true for different types of interrelated chemicals. These ontologies will be essential to creating the smart search and browsing capabilities that scientists in the CHEAR program will have access to.
The same ontology-enabled data service approach is being developed by McGuinness’ team along with materials scientists for the nanocomposite data community and now has expanded to include additional types of materials with a new award for meta materials as part of the NSF Harnessing the Data Revolution program.
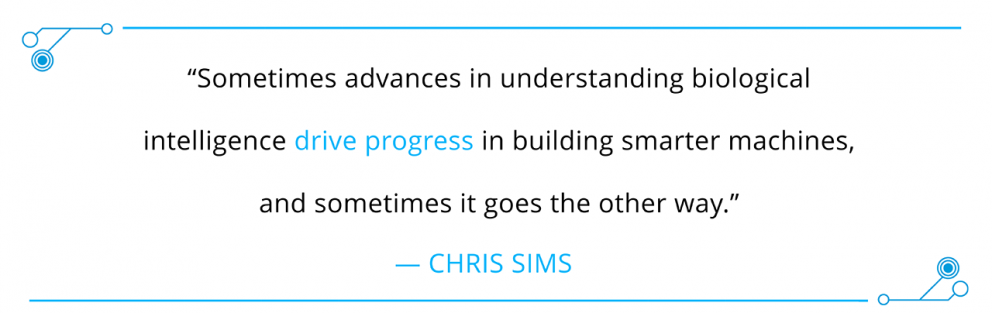
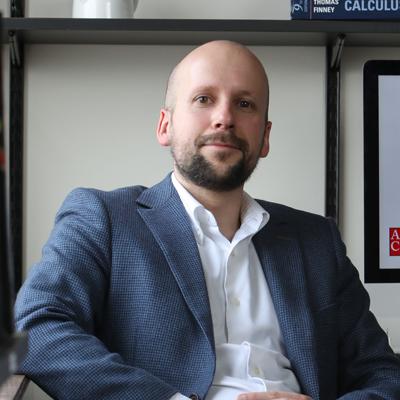
Reinforcement Learning
In 2016, an artificial intelligence company developed a computer program that was able to beat a human grand master in the abstract strategy game of Go. It did this by combining neural networks and “reinforcement learning,” an approach that mimics how biological systems learn from their past experience in order to improve behavior.
A similar system was able to learn to play a suite of classic Atari 2600 video games. While it excelled at some games, it was bad at others. It could dominate in Pong, for example, but could be outperformed by even novice human players in Ms. Pac-Man.
What accounts for this mixed bag? According to Chris Sims, assistant professor of cognitive science, what is missing is a computational theory of generalization that can guide us in building AI systems that learn better from their past experience.
“While neural networks and reinforcement learning are able to show some forms of generalization, we don’t really have a deep scientific basis for understanding how past experience should extrapolate to new situations,” he says.
In a 2018 paper in Science, Sims took a step toward solving this problem by bridging two disparate fields: Information Theory, a branch of mathematics that underlies modern communications technology by making it possible to predict the best possible performance of an artificial communication system given the limits of the system, and the Universal Law of Generalization, a canonical law of cognitive science that describes the likelihood that a biological system will extend a past experience to new stimulus.
By combining these two approaches, Sims developed a computational theory of how intelligent systems should generalize. His work successfully explained a wide range of classic psychology experiments on human generalization. The goal now is to turn this same approach toward improving the generalization abilities of AI.
Exploiting the bridge he discovered, Sims is developing new reinforcement learning algorithms to reflect theories of biologically inspired generalization. In a small-scale demonstration, Sims trained an AI system to navigate a “grid world” — the digital equivalent of a mouse running a maze in order to find a piece of cheese.
After training the artificial agent, he changed the maze by adding or moving walls. Solving the modified maze requires a basic form of generalization, because previous routes to the goal might now be blocked. Sims showed that his new algorithms were able to generalize better compared to standard approaches to reinforcement learning. His work provides a theoretical understanding of how and why the systems were able to better extend their past experience to new environments.
He’s currently working on scaling this work up to larger and larger domains, such as the suite of Atari video games that has proven so challenging for past AI systems.
“AI and cognitive science have a long history of leapfrogging each other,” Sims says. Reinforcement learning, for example, originally grew out of research on animal learning.
“Sometimes advances in understanding biological intelligence drive progress in building smarter machines, and sometimes it goes the other way. The key to progress,” he says, “is the ability to recognize, and combine, good ideas from across widely different fields.”